ABSTRACT.- Early detection and monitoring of invasive forest pests are crucial for effective pest management, particularly in preventing large-scale damage, reducing eradication costs, and improving overall control effectiveness. This study investigates the potential of machine learning models and remote sensing at various spatiotemporal scales to assess forest damage caused by the woodwasp Sirex noctilio in pine plantations. A Random Forest (RF) model was applied to analyze Planetscope satellite images of Sirex-affected areas in Neuquén, Argentina. The model's results were validated through accuracy analysis and the Kappa method to ensure robustness. Our findings demonstrate that the RF model accurately classified Sirex damage levels, with classification accuracy improving progressively over time (overall accuracy of 87% for five severity categories and 98% for two severity categories). This allowed for a clearer distinction between healthy and Sirex-infested trees, as well as a more refined categorization of damage severity. This study highlights the potential of machine learning models to accurately assess tree health and quantify pest damage in plantation forests, offering valuable tools for large-scale pest monitoring. © 2025 by the authors. Licensee MDPI, Basel, Switzerland.
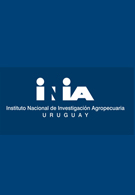