The main goal of researchers in genetic breeding programs is to select superior genotypes and recommend varieties through effective selection methods. Thus, the objective of this study was to evaluate the performance of Artificial Neural Networks (ANN) in predicting genetic values for progeny selection of Eucalyptus sp. For the training of ANN, 64 experiments were simulated that varied among means (5, 10, 15 and 20), heritability (10, 20, 30 and 40%) and coefficient of variation (10, 20, 30 and 40%). For validation of ANN, data from a progeny test of Eucalyptus camaldulensis was used. The genetic values of both the simulated and progeny data were obtained by the REML / BLUP procedure. The ANN used was a multiple layer type with three inputs (phenotype value, block means, and progeny mean), a hidden layer containing four neurons and one exit layer. The algorithm used was backpropagation. The correlation between genetic values predicted by the BLUP methodology and those obtained by ANN was 99% in the training phase and 91% in the validation stage. The good performance of ANN in the validation stage reflected in the correlation of the ordering of individuals (92%) and families (99%) of E. camaldulensis by the two methods. Thus, multiple layer ANN showed good performance in predicting genetic values in progeny tests of Eucalyptus sp. for DBH which are promising tools for selection of progenies in forest breeding programs.
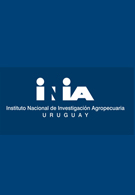