ABSTRACT.- Several studies have used aerial images to predict physiological maturity (R8 stage) in soybeans (Glycine max (L.) Merr.). However, information for making predictions in the current growing season using models fitted in previous years is still necessary. Using the Random Forest machine learning algorithm and time series of RGB (red, green, blue) and multispectral images taken from a drone, this work aimed to study, in three breeding experiments of plant rows, how maturity predictions are impacted by a number of factors. These include the type of camera used, the number and time between flights, and whether models fitted with data obtained in one or more environments can be used to make accurate predictions in an independent environment. © 2024 by the authors. Licensee MDPI, Basel, Switzerland.
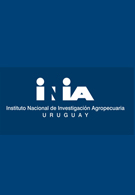